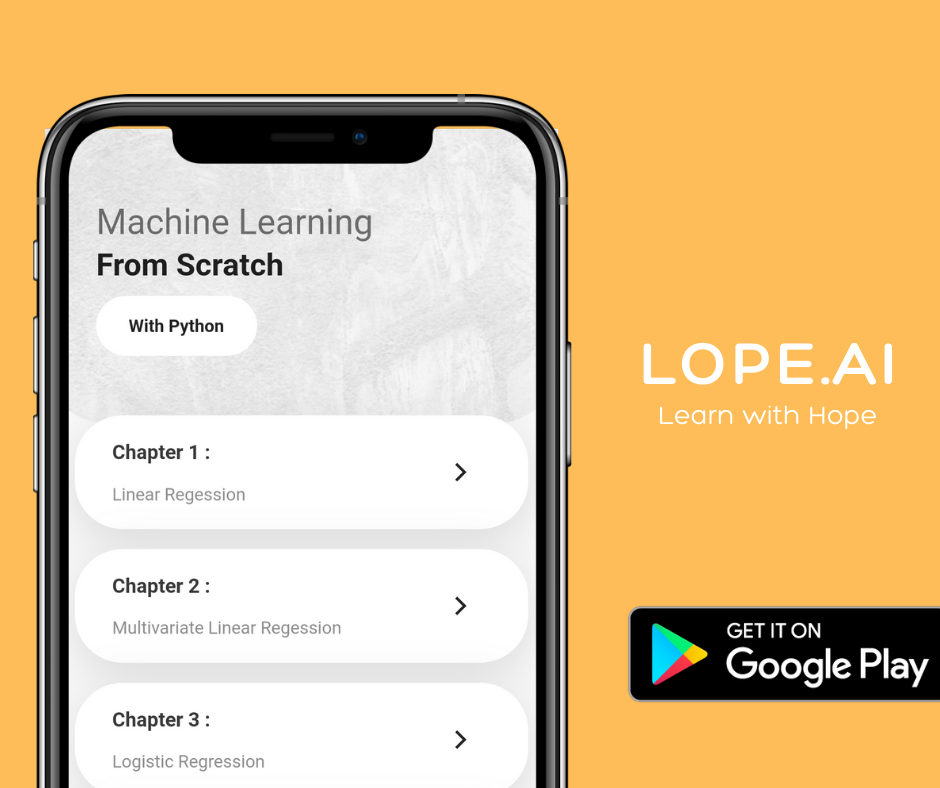
update : 機械学習/AI用の対話型学習アプリを導入しました。 >> Check it out for Free now <<
Import the required libraries
import numpy as np
import pandas as pd
eps = np.finfo(float).eps
from numpy import log2 as log
‘eps’ here is the smallest representable number.この数値は最小の数値です。 分母にlog(0)や0が入ることがありますが、それを避けるためにこれを使用します。
データセットの定義:
Create pandas dataframe :

さて、決定木作成手順を覚えておきましょう・・・・・・。
1.compute the entropy for data-set2.for every attribute/feature:
1.calculate entropy for all categorical values
2.take average information entropy for the current attribute
3.calculate gain for the current attribute3. pick the highest gain attribute.
4. Repeat until we get the tree we desired
- データセットを分割する際のエントロピーと情報利得を求める。
クラス(対象変数ベクトル)を取り込んでそのクラスのエントロピーを求める関数を定義しておきます。
ここで分数は「π」であり、分割前のグループ(親グループ)の要素数に対する分割後のグループの要素数の割合です。

2 で得たのと一緒です。
各属性のエントロピーを名前と一緒に格納します。 各属性のInfo Gainを計算する :
IG (infogain) を計算する関数を定義する :
IG(attr) = entropy of dataset – entropy of attribute
def ig(e_dataset,e_attr):
return(e_dataset-e_attr)
各attrのIGをdictに格納する :
#entropy_node = entropy of dataset
#a_entropy = entropy of k(th) attrIG = {k:ig(entropy_node,a_entropy) for k in a_entropy}

見ての通りoutlookが最も情報量が多く0.1であることがわかる。4757>

さて、木を進めるために再帰を使用します
木を得るまでサブツリーに対して同じことを繰り返してください。

これに基づいて決定木を構築するんだよ。 以下は完全なコードです。

visit Pytholabs.com for amazing courses
を参照してください。